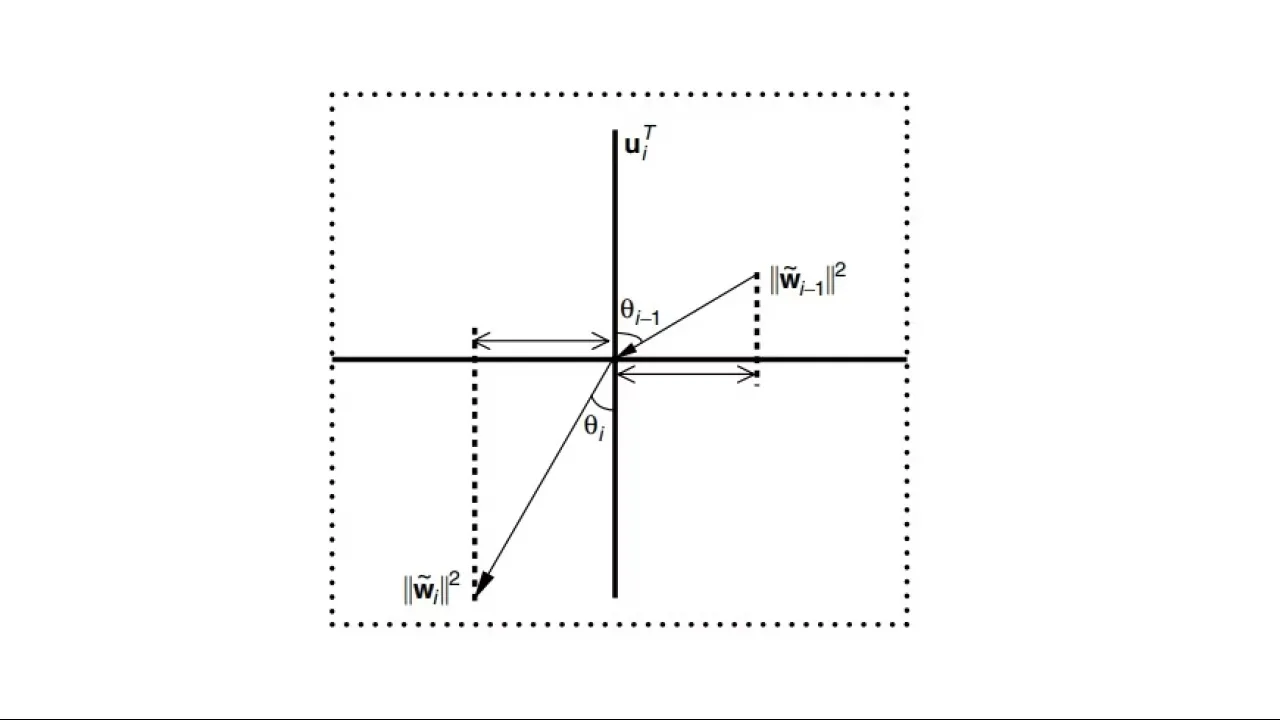
Energy Conservation in Adaptive Filtering
About
A. H. Sayed, Tareq Y. Al-Naffouri, and Vitor H. Nascimento, "Energy conservation in adaptive filtering", Nonlinear Signal and Image processing: Theory, Methods, and Applications, CRC Press, November 2003.
Introduction:
The study of the steady-state and transient performance of adaptive filters is a challenging task because of the nonlinear and stochastic nature of their
update equations (e.g., References 1 to 4). The purpose of this chapter is to provide an overview of an energy-conservation approach to study the performance of adaptive filters in a unified manner.4 The approach is based on showing that certain a priori and a posteriori errors maintain an energy balance for all time instants.(5–7) When examined under expectation, this energy balance leads to a variance relation that characterizes the dynamics of an adaptive filter.(10–14) An advantage of the energy framework is that it allows us to pushthe algebraic manipulations of variables to a limit, and to eliminate unnecessary cross-terms before appealing to expectations. This is a useful step because it is usually easier to handle random variables algebraically than under expectations, especially for higher-order moments. A second advantage of the energy arguments is that they can be pursued without restricting the distribution of the input data. To illustrate this point, we have opted not to restrict the regression data to Gaussian or white in most of the discussions below. Instead, all results are derived for arbitrary input distributions. Of course, by specializing the results to particular distributions, some known results from the literature can be recovered as special cases of the general framework. As for most adaptive filter analysis, progress is difficult without relying on simplifying assumptions. In the initial part of our presentation, we derive exact energy-conservation and variance relations that hold for a large class of adaptive filters without any approximations. Subsequent discussions will call upon simplifying assumptions to make the analysis more tractable. The assumptions tend to be reasonable for small step sizes and long filters.